Blackbox Machine Learning
Updated on 02.04.25
5 minutes to read
Copy link
Overview
Rely on SEON's blackbox machine learning algorithm to crunch the numbers behind the scenes and prevent fraud that human eyes are unlikely to catch. Our Blackbox models calculate Fraud probability scores, which are separate from a transaction's SEON Fraud Score, to give you yet another indicator of the risks associated with it. Read below to get the most out of our Blackbox models such as Blackbox Score, Email Network Score and Phone Network Score.
SEON's machine learning tools
Glance at our different machine learning tools to understand how they differ.
Whitebox | Blackbox | |
Automatic retraining | Yes | Yes |
Uncovers complex fraud patterns | Yes | Yes |
Human-readable rules | Yes | No |
Transparent decision-making | Yes | No |
Automatic rule creation | Yes | No |
Can be used in rules | No | Yes |
Can effect fraud score | No | Yes |
Can change transaction state | Yes | Yes |
Fraud probability scoring | No | Yes |
Keeps you in control | Yes | Yes |
Fully automatable as needed | Yes | Yes |
Available from Day 1 | No* | Yes |
* Whitebox machine learning requires account-specific training data to begin recommending rules: at least 1000 transactions with 100 in the DECLINE and 100 in the APPROVE state.
Using blackbox machine learning
Blackbox machine learning will not directly affect a transaction's SEON fraud score. Unlike our whitebox model, it will not recommend human-readable rules that you can enable. However, the Blackbox score represents a standalone fraud probability metric incorporating all aspects of data SEON processes about a transaction.
Blackbox score
The blackbox model calculates a fraud probability score, known as the blackbox score, on a scale between zero (0) and one hundred (100). You can use the blackbox score to make decisions automatically or create custom rules that influence the overall fraud score based on it.
While called the blackbox model, our solution is actually explainable AI. However, the details of how the blackbox model reaches its conclusion and presents a given score cannot be seen on the Admin panel.
Our data scientists can create a report on the most important features of the blackbox score calculation upon request. This report will explain key correlations identified in the data used by the AI to create the blackbox score. For example, the blackbox score usually decreases when the number of a user's social and digital profiles increases.
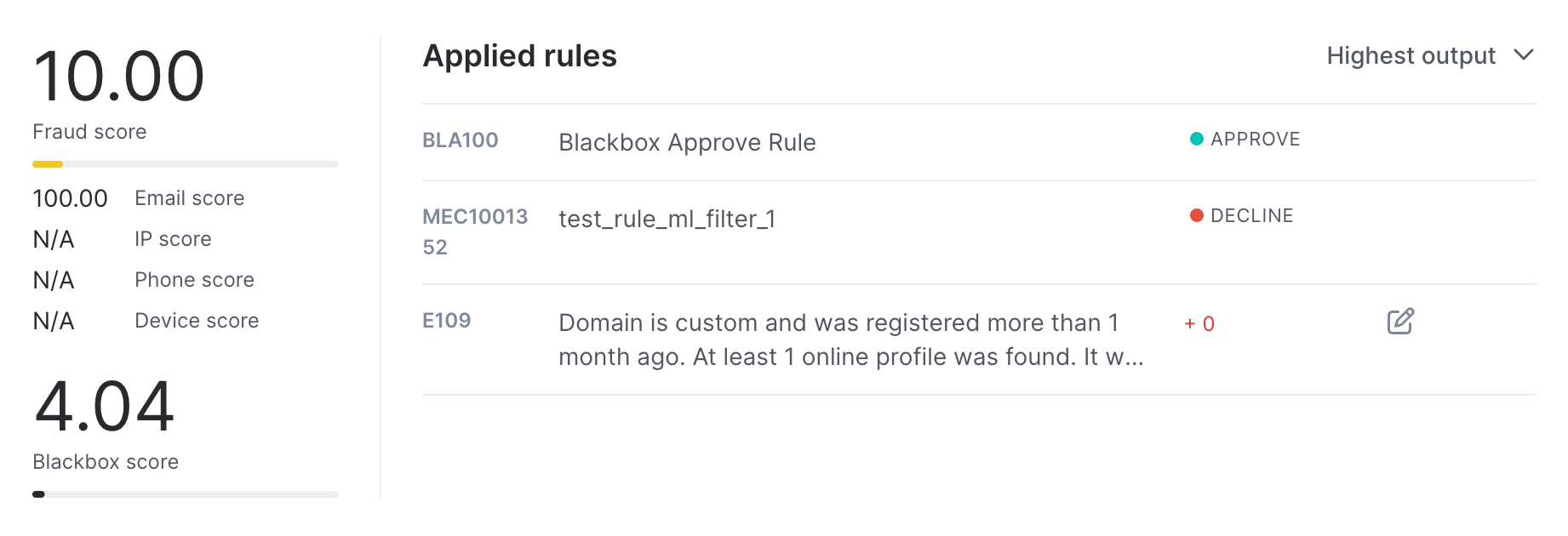
The blackbox score is displayed on the Applied rules widget of the Transaction details page.
The model may use parameters and correlations that humans cannot spot easily, and it retrains itself daily. As a result, it helps you stay ahead of complex fraud schemes and change tactics with ease.
Use the blackbox score as a second layer of defense or an indicator of the risk associated with a transaction independent of your rules. You can also fully automate fraud-fighting by relying on the blackbox score.
Base model and custom model
When you first start using SEON, your account will likely not contain enough custom data for the blackbox model to work efficiently. However, thanks to our base model, you can benefit from its speed and accuracy from day one.
The base model is a generic anonymized collection of transactions encountered by SEON that we use to train the Blackbox Machine Learning algorithm. The base model turns off when your account passes 1000 transactions, with at least 100 in the DECLINE state and 100 in the APPROVE state. Once you have passed this threshold, the Blackbox model will train on your unique data.
Retail Blackbox score: Using blackbox fraud likeliness score in retail and e-commerce businesses.
Fraud patterns vary across different industries, and geographical locations play a crucial role in retail. Products are shipped to specific places, users are located in certain areas, and billing regulations are tied to locations. As a retail or e-commerce business using SEON, we provide a specialized version of the Blackbox score that considers these industry-specific fraud patterns.
To maximize the effectiveness of SEON Blackbox’s fraud likelihood score in retail, enable the Geolocation Details capabilities. These capabilities calculate the distances between the user’s, shipping, and billing addresses. Contact the SEON team to enable this feature.
With geolocation details enabled, the Blackbox Score automatically incorporates these distance fields into the customer-specific model, which is trained to adapt to retail fraud patterns. This makes our model a Retail-Blackbox model. We recommend using Geolocation Details in retail. Our team will assist you in enabling this feature and discuss its minor impact on SEON’s overall response time to ensure the best setup for your needs.
Network Scores
Advanced Digital Footprinting (ADF) extends beyond raw data by introducing check aggregates and abstracted data points, which help stabilize API responses and reduce error rates. The solution also incorporates consortium data into SEON’s proprietary network risk scores, offering a faster, more convenient, all-in-one risk assessment. This section explains the value of network scores in providing reliable risk indications.
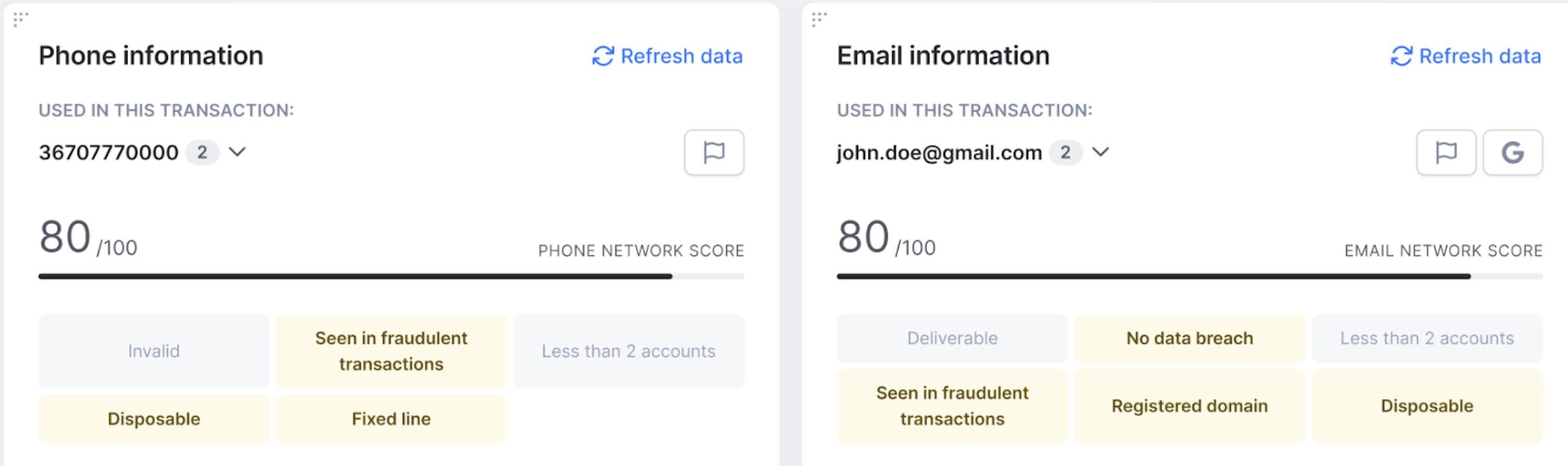
Like SEON’s Blackbox Score, Network Scores are calculated through machine learning models. These models capture parameters and correlations that are often difficult for humans to detect. SEON’s Email and Phone Network Score models, for example, rely on specific subsets of data, blending email or phone data with SEON’s unique consortium data. The resulting Network Scores produce an email fraud-likelihood score (Email Network Score) and a phone fraud-likelihood score (Phone Network Score), both scaled between 0 and 100.
When you start using SEON, your account may initially lack enough customer data for Network Score models to operate at full capacity, similar to how the Blackbox Score model functions. However, with our Network Score base models, you’ll still benefit from reliable speed and accuracy right from day one. Once your account surpasses the threshold of eligible labeled transactions, the Network Score models will begin training on your unique data for enhanced accuracy.
Blackbox settings
The Machine Learning tab of the Settings page houses all settings related to your whitebox and blackbox models.

Use the top-most toggle to enable or disable Blackbox scores for your account. Use this setting to monitor these scores without them affecting your transactions automatically.
To automate fraud prevention using the blackbox model enable the Approve transactions below a certain Blackbox score and Decline transactions above a certain Blackbox score toggles.
A slider will appear for you to set the thresholds according to your risk appetite. Feel free to adjust these values at any time, based on your results with blackbox machine learning.
Learn more
Read more about how SEON harnesses the power, speed and accuracy of machine learning and what you can do to get the best results.